Tenstorrent Launches Wormhole AI Processors: 466 FP8 TFLOPS at 300W
by Anton Shilov on July 19, 2024 2:30 PM EST- Posted in
- AI
- Tenstorrent
- Wormhole
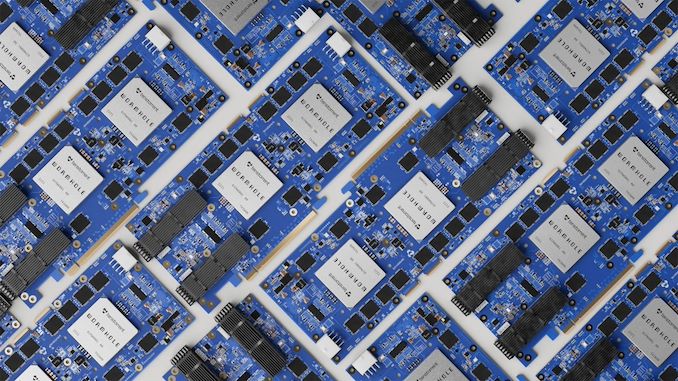
Tenstorrent has unveiled its next-generation Wormhole processor for AI workloads that promises to offer decent performance at a low price. The company currently offers two add-on PCIe cards carrying one or two Wormhole processors as well as TT-LoudBox, and TT-QuietBox workstations aimed at software developers. The whole of today's release is aimed at developers rather than those who will deploy the Wormhole boards for their commercial workloads.
“It is always rewarding to get more of our products into developer hands. Releasing development systems with our Wormhole™ card helps developers scale up and work on multi-chip AI software.” said Jim Keller, CEO of Tenstorrent. “In addition to this launch, we are excited that the tape-out and power-on for our second generation, Blackhole, is going very well.”
Each Wormhole processor packs 72 Tensix cores (featuring five RISC-V cores supporting various data formats) with 108 MB of SRAM to deliver 262 FP8 TFLOPS at 1 GHz at 160W thermal design power. A single-chip Wormhole n150 card carries 12 GB of GDDR6 memory featuring a 288 GB/s bandwidth.
Wormhole processors offer flexible scalability to meet the varying needs of workloads. In a standard workstation setup with four Wormhole n300 cards, the processors can merge to function as a single unit, appearing as a unified, extensive network of Tensix cores to the software. This configuration allows the accelerators to either work on the same workload, be divided among four developers or run up to eight distinct AI models simultaneously. A crucial feature of this scalability is that it operates natively without the need for virtualization. In data center environments, Wormhole processors will scale both inside one machine using PCIe or outside of a single machine using Ethernet.
From performance standpoint, Tenstorrent's single-chip Wormhole n150 card (72 Tensix cores at 1 GHz, 108 MB SRAM, 12 GB GDDR6 at 288 GB/s) is capable of 262 FP8 TFLOPS at 160W, whereas the dual-chip Wormhole n300 board (128 Tensix cores at 1 GHz, 192 MB SRAM, aggregated 24 GB GDDR6 at 576 GB/s) can offer up to 466 FP8 TFLOPS at 300W (according to Tom's Hardware).
To put that 466 FP8 TFLOPS at 300W number into context, let's compare it to what AI market leader Nvidia has to offer at this thermal design power. Nvidia's A100 does not support FP8, but it does support INT8 and its peak performance is 624 TOPS (1,248 TOPS with sparsity). By contrast, Nvidia's H100 supports FP8 and its peak performance is massive 1,670 TFLOPS (3,341 TFLOPS with sparsity) at 300W, which is a big difference from Tenstorrent's Wormhole n300.
There is a big catch though. Tenstorrent's Wormhole n150 is offered for $999, whereas n300 is available for $1,399. By contrast, one Nvidia H100 card can retail for $30,000, depending on quantities. Of course, we do not know whether four or eight Wormhole processors can indeed deliver the performance of a single H300, though they will do so at 600W or 1200W TDP, respectively.
In addition to cards, Tenstorrent offers developers pre-built workstations with four n300 cards inside the less expensive Xeon-based TT-LoudBox with active cooling and a premium EPYC-powered TT-QuietBox with liquid cooling.
Sources: Tenstorrent, Tom's Hardware
20 Comments
View All Comments
GeoffreyA - Friday, July 19, 2024 - link
Excellent names! Wormhole and Blackhole.PeachNCream - Sunday, July 21, 2024 - link
Yeah, accurate too since humanity is sending an awful lot of power into the blackhole of AI. MS and Alphabet/Google both noted AI energy consumption has significantly increased their reliance on burning non-renewables and we're currently as a planet, consuming whole nation states' worth of power to run things we keep telling ourselves constitute AI but are really not independently intelligent.I suppose the alternative is letting foolish people drive around in excessively large personal transport vehicles and run 800+W computing devices for the sole purpose of playing video games. At least we're not doing that too...oh wait, nevermind.
GeoffreyA - Sunday, July 21, 2024 - link
All that matters to these corporations is making dollars, and at present, the road to riches is AI Street. So what if an inordinate amount of power is used in the process? We'll sweep that under the carpet and later greenwash it. "Because we care" (audience applauding).On a serious note, I think the exorbitant energy use of current deep neural networks is a barrier (downplayed of course). It's far behind the brain in this regard. Nature chose a biological, analogue implementation, using far less energy and doing much more. I do not doubt the breakthrough will use such an approach, getting ever nearer to the brain's efficiency. These are the MS-DOS versions of Windows 10.
boozed - Sunday, July 21, 2024 - link
They *think* the road to riches is AI. It's eating amazing amounts of VC and could very well turn out to be a black hole.GeoffreyA - Monday, July 22, 2024 - link
Exactly, they *think* it is. It could well fizzle away before we know it. Maybe cloud will make a return to the limelight.Santoval - Friday, July 26, 2024 - link
Neuromorphic processors have existed for about a decade now. They are about a thousand times more energy efficient, massively parallel, and lack a Von Neumann bottleneck since logic memory and I/O are all tightly intertwined - much like in the human brain.Their energy efficiency and complexity lies somewhere in the middle between primate brains and Von Neumann processors, and they look like the hardware analogue of software AI/LLMs.
So they should be ideally suited for them. And yet every AI company uses Nvidia's obscenely energy inefficient GPUs. Why? Due to addiction to CUDA?
GeoffreyA - Saturday, July 27, 2024 - link
Thanks for the description. You've given me something to read about over the weekend. The concept of neuromorphic processors is what I was vaguely thinking of. It seems remarkable to me that the technology exists---I had thought it decades away---but is being overlooked in favour of the same-old way of thinking. As for CUDA, it is regrettable that so many machine-learning libraries rely on it, and is in nobody's interest but Nvidia's.I would be glad to see neuromorphic processors gaining traction. I believe today's LLMs are the primitive analogues of parts of our brain. If these could be extended, joined with the neuromorphic system, and consciousness cracked, doubtless it would lead to strong AI. Put differently, I think we are the same system but at an advanced stage.
Santoval - Saturday, July 27, 2024 - link
An additional reason for their very slow adoption might be that they have a different programming paradigm, which AI developers are not familiar with. There are no conventional back & forths between memory and logic after all.Imagine *all* memory being at the L0 cache / register level of conventional processors and being just as fast. It doesn't look like OpenAI, at least, is comfortable with their dependence on Nvidia. Otherwise they would not have developed Triton to compete with CUDA.
But Triton is still at an early stage of development, and OpenAI initially released it only with Nvidia support. But if it takes off it will be a viable competitor to CUDA, and cross-platform too.
Despite being Python like OpenAI claims it's at least just as fast as CUDA.
The only other cross platform solution is OpenCL, but how many use that for AI vs CUDA?
GeoffreyA - Saturday, July 27, 2024 - link
Machine learning is hard as it is and programmers are only now coming to terms with it. A new paradigm needs time and major benefits, and the present approach is the path of least resistance.I doubt whether, but hope, Triton will usurp CUDA's place. We need a real competitor, that's for sure. CUDA was just too good and accessible: Nvidia built the ecosystem, the libraries, the tools, and everything was just there and ML began gravitating towards it. OpenCL seems to be going the way of the dinosaur: one doesn't see it much any more. I have seen Vulkan used, in Real-ESRGAN, but this isn't common. There is a library that translates CUDA for AMD and Intel GPUs but is neither complete nor the answer.
charlesg - Monday, July 22, 2024 - link
Last I checked, it's a free world.One large difference between you and I, is I couldn't care less about what you think or do.
Unless you desire to force your distorted viewpoint on others, which based on your regular posts, appears that way.